Is AI’s hype over — or is a new computing era just beginning?
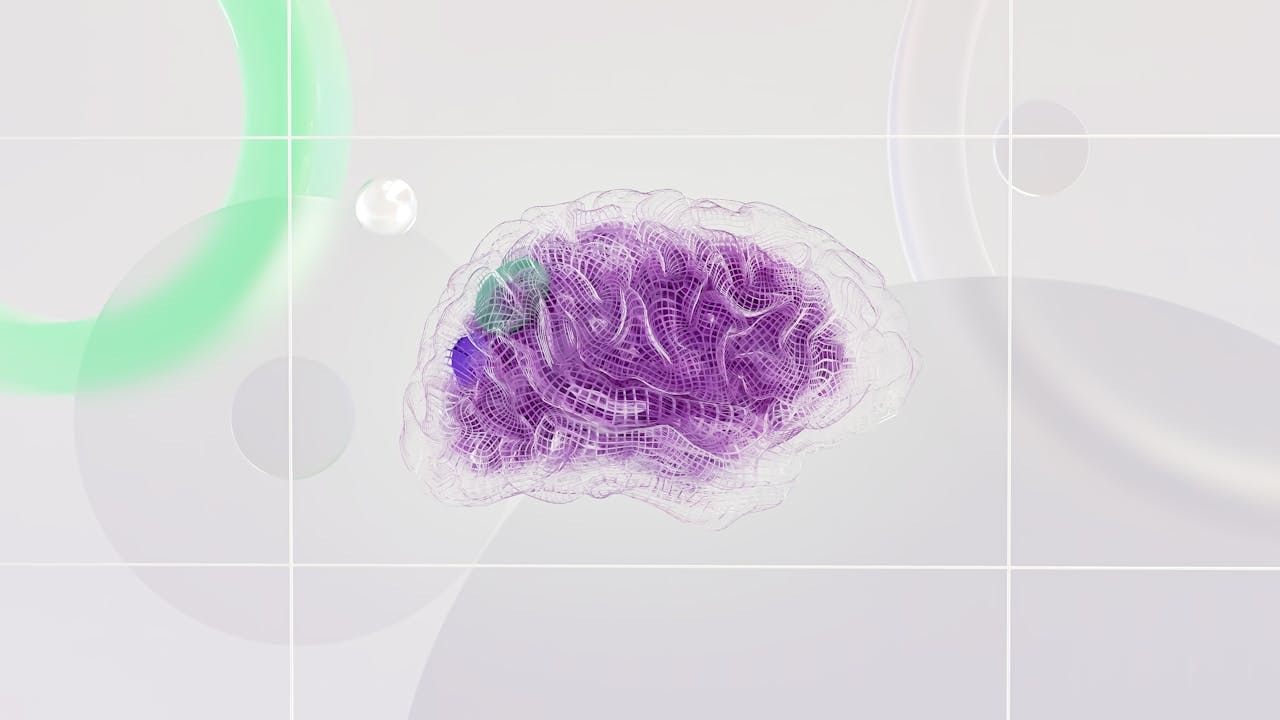
by Radix Ventures Team
The limits of today’s large language models
Generative AI leapt from lab demo to boardroom agenda in record time, but the real-world applications revealed real flaws. Hallucinations, shallow reasoning, and “context amnesia” show that current large language models (LLMs) are statistical parrots, not crystal-clear oracles. Token ceilings mean a multi-hour chat quickly drifts off topic, while heavyweight training pipelines strain data supplies and power grids. Nail these pain points and you hear the familiar verdict: “LLMs have peaked; time to move on.”
Yet each weakness is a door, not a dead end. Retrieval-augmented generation reduces hallucinations by allowing models to look things up instead of making them up. Memory-extended architectures, such as Claude 3’s 200k-token window and emerging million-token research prototypes, keep conversations coherent. And the world is hardly short of data, just short of structured data. Multimodal sensors, synthetic simulation logs, and user feedback are opening new, endless sources of high-quality training material.
Why the curve is set to bend upward
If the internet era taught us anything, it’s that scaling curves bend when architecture changes. The jump from single-core CPUs to many-core GPUs sparked today’s AI explosion; the shift from text-only LLMs to multimodal, tool-using systems sets up the next. Three shifts are already visible:
- Vision-Language Models (VLMs). By fusing images, video, and text, these models can draft marketing copy from a whiteboard sketch or reverse-engineer a schematic posted on Slack. They widen the data lens and multiply commercial touch points. Most frontier models now launch as multimodal.
- Smaller and smarter models. Techniques such as low-rank adaptation (LoRA), mixture-of-experts (MoE) routing, and speculative decoding cut inference cost by an order of magnitude, making fine-tuned models run on a laptop or a factory edge server. That flips the economic equation from “rent a GPU” to “embed an assistant everywhere.”
- Long-horizon reinforcement learning. Instead of predicting the next token, frontier models learn to plan across hundreds of steps. That capability underpins autonomous agents that can manage IT tickets, optimize supply chains, or pilot a robot arm.
Two tracks for tomorrow’s AI
Agentic AI – think of self-directed software employees. Give an agent a goal (“Find 50 high-fit prospects”); it chooses tools, writes code, iterates, and delivers a report while you sleep. Early versions, such as AutoGPT, initially appeared to be gimmicks, but the principle is now integrated into Microsoft Copilot, Google Workspace, and a wave of vertical SaaS solutions. Every business workflow containing a loop of “search, transform, write” is a candidate for full or partial autonomy.
Physical AI – the crossover with robotics and digital twins. Training on high-fidelity simulations, such as those inside NVIDIA Omniverse, enables models to learn the physics of conveyor belts, reagents, or parking lots, and then transfer the policy to a real robot. The same pipeline lets energy developers test carbon-capture plants entirely in silico before pouring concrete. It can also generate millions of hours of edge-case training data for autonomous vehicles—for example, simulating a child chasing a ball and suddenly darting into the street. When a model understands both language and force vectors, you get systems that can read a maintenance manual and turn the wrench.
Infrastructure: the less glamorous, trillion-dollar upside
Running an LLM query costs cents; running a swarm of agents or a fleet of robots costs square meters of silicon and megawatts of power. That creates investable pressure across the stack:
- Hardware – NVIDIA’s Blackwell GPUs, Google’s TPUs, and a crop of wafer-scale startups are targeting 10× performance-per-watt improvements by 2027. Custom inference ASICs aimed at small models may become the new edge GPU.
- Data center infrastructure, interconnects – As model shards span thousands of accelerators, network fabrics—such as NVLink, Ethernet with RoCE, and photonics—become a gating factor, not an afterthought.
- Software – New models introduce new layers and operations, all of which need to be highly optimized for the existing stack. This requires a significant workload of highly skilled performance engineers. Optimizations are also essential to overall data center level efficiency, NVIDIA kicked off the Dynamo, i.e., project, where they create an “operating system” for a datacenter of multiple inference endpoints.
- Developer tooling – Agents need observability, safety baffles, and orchestration APIs. We expect the “Kubernetes moment” for AI within two years, spawning a platform layer that abstracts away hardware quirks and compliance headaches.
- Energy – Data-center demand is forecast to double by 2030, with AI seen as the prime driver. Expect a land grab for cheap renewables and a resurgence of on-premises “micro data centres” colocated with industrial waste heat or small modular reactors. Moving to edge computing will also partially help with this problem.
What does this mean for investors
Value in the LLM layer is consolidating around a few foundation-model vendors, but the application and infra layers remain wide open. We see at least the following three sweet spots.
- Agent verticals – domain-tuned agents that own data and workflow in verticals with structural labor shortages or high labor intensity (accounting, insurance claims, biomedical R&D).
- Simulation tools – software that feeds physical AI with digital twins, scenario libraries, and synthetic data pipelines, including manufacturing, robotics, or autonomous vehicles.
- AI operations – security, cost-control, and compliance platforms that make enterprise AI reliable and sustainable.
The open question is unit economics, i.e., does the product deliver at least a 10× improvement in speed or cost after factoring in the hardware spend? Teams that treat compute and data as first-class citizens—optimizing prompts, pruning parameters—will outcompete those chasing raw model size.
In summary, we are at the end of the beginning
LLMs’ shortcomings are real, but they are childhood illnesses, not terminal conditions. The stack is already mutating to address them, while entirely new modes—multimodal perception, long-horizon planning, embodied action—are coming online. That is what a platform transition looks like: messy in phase one, exponential in phase two.
For investors and founders, the takeaway is clear. Don’t write AI off as yesterday’s fad; write the checks that build the infrastructure and applications powering its next decade. Hype dies; platforms endure.
Explore More Insights and Stories
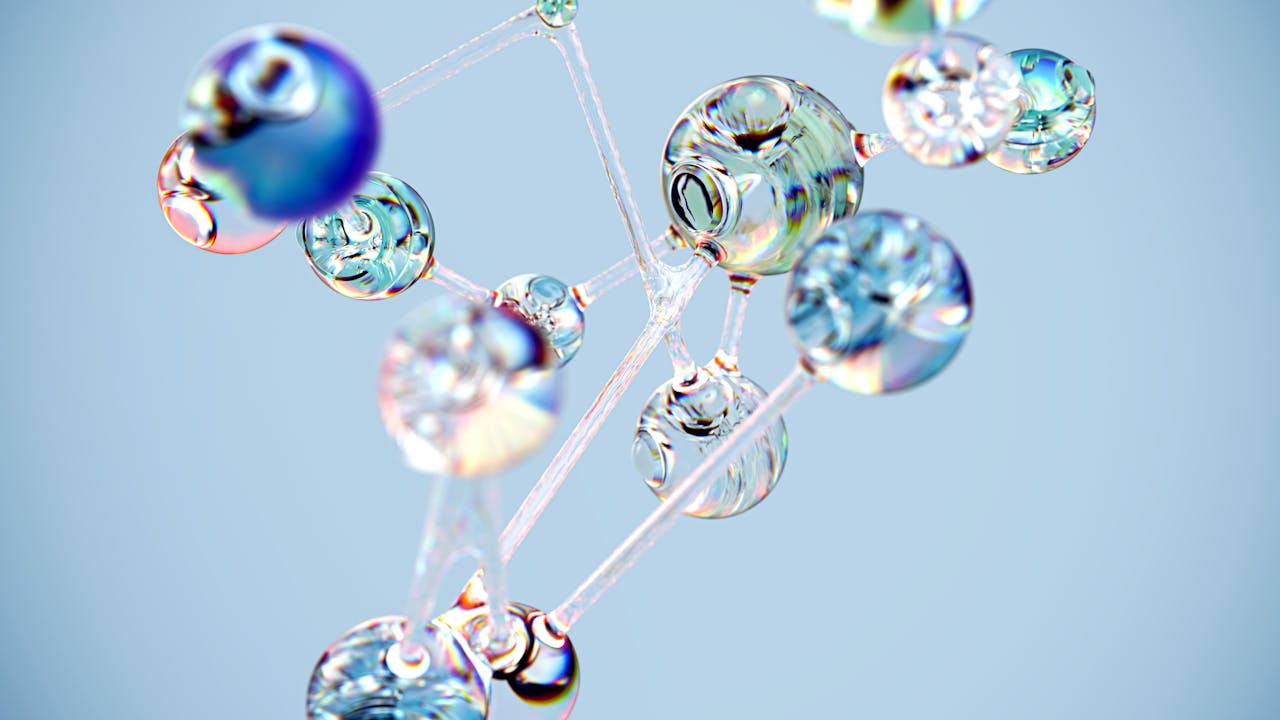
A tiny revolution: Exploring the world of nanotechnology
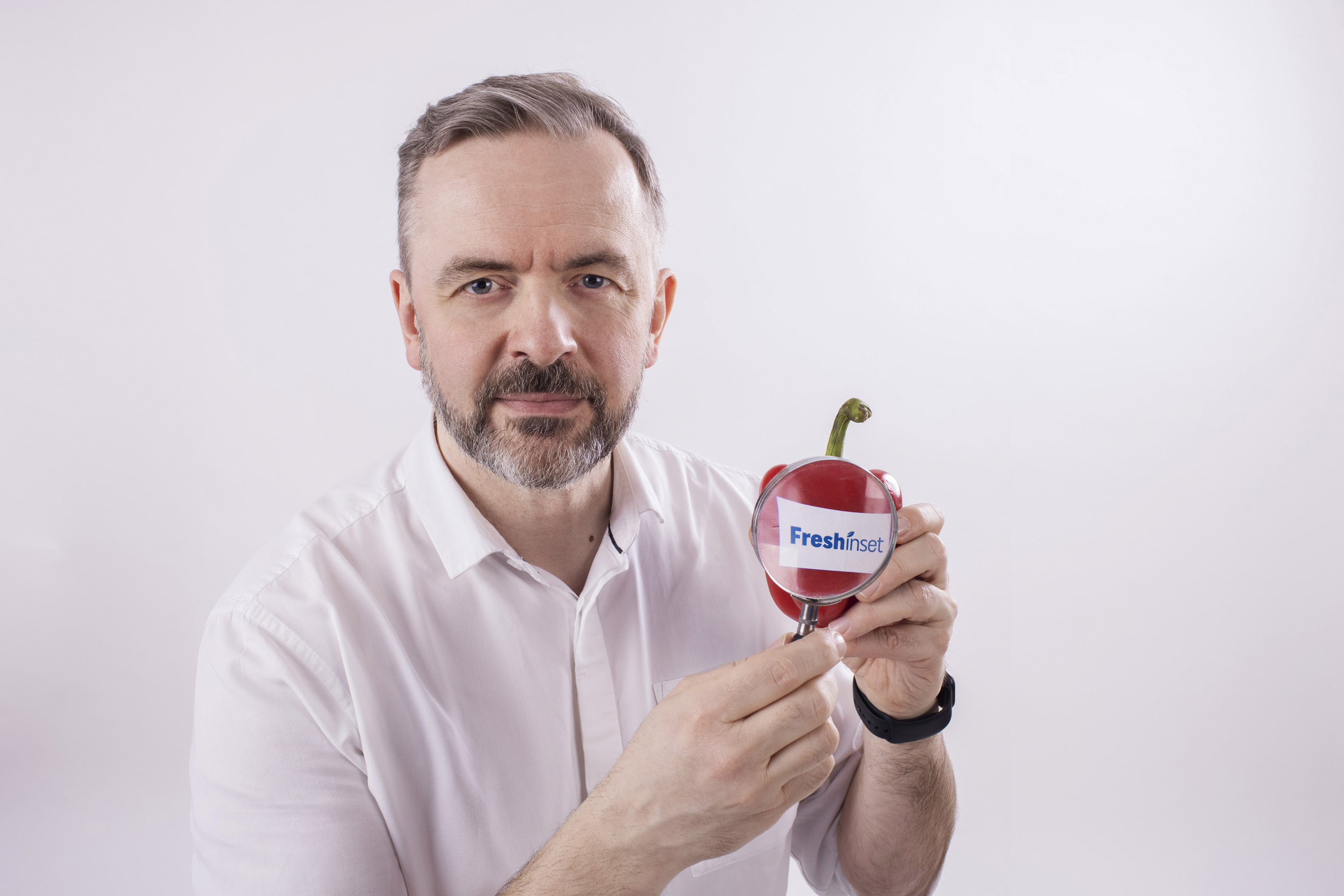
Radix Ventures invests €1.5M in Fresh Inset, supporting cutting-edge freshness technology for fruits, vegetables and flowers.
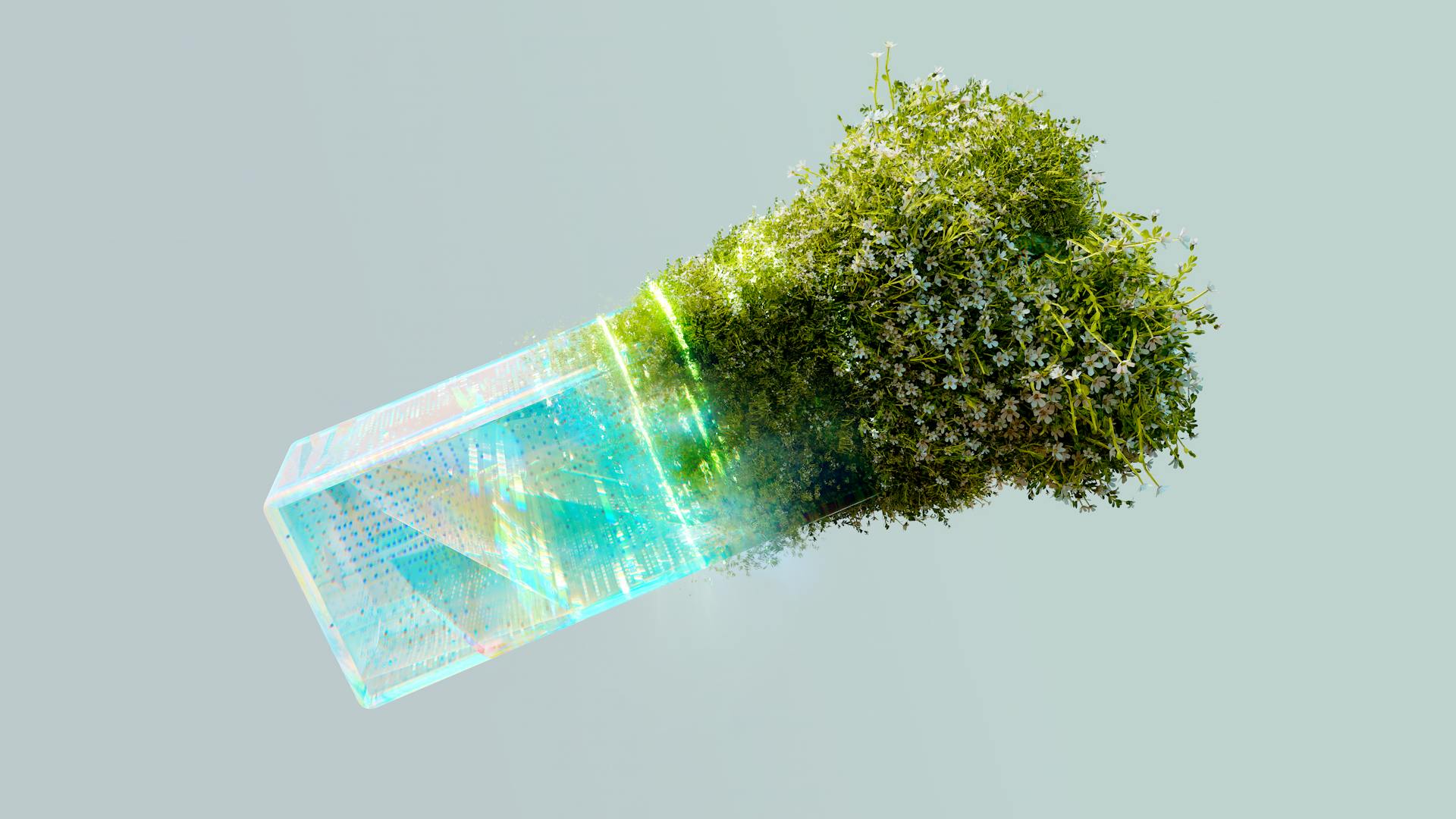